NMSU computer science professor, students co-author article published in Nature Methods
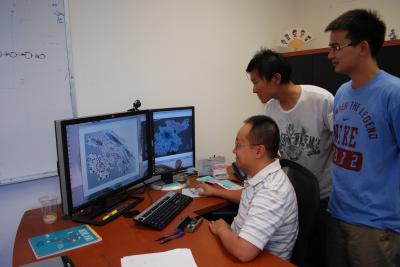
Joe Song, along with his graduate students Yang Zhang and Haizhou Wang, and former NMSU student Zhengyu Ouyang, are co-authors of "Wisdom of crowds for robust gene network inference," the result of a long-standing challenge in systems biology - to uncover and model gene regulatory networks.
Through the Dialogue on Reverse Engineering Assessment and Methods (DREAM5) Network Inference Challenge, researchers around the world performed a comprehensive blind assessment of more than 30 network inference methods on Escherichia coli, Staphylococcus aureus, Saccharomyces cerevisiae and in silico microarray data to see which method performed the best. They characterized the performance, data requirements and inherent biases of different approaches, and provided guidelines for algorithm development.
"In this paper, we show that the best performing method is the one that combines all the results from different groups," Song said. "No single method stands out."
One cannot yet directly videotape dynamic interactions in gene networks. What scientists can see are the snapshots of concentration of molecules.
In networks of thousands of genes, Song said they look to see how genes are associated with each other. If genes change together, that indicates potential interactions between them.
"The map of such connections enable us to understand how a biological system functions," Song said. "This will tell us, for example, key genes that regulate the expression of proteins in a tumor cell. And this eventually can offer an insight on the cell's behavior."
This research, Song said, is going to bring many opportunities in the future. This particular phase of the challenge exposed the disadvantages of the various methods and showed there are still technical barriers to dealing with real biological networks, since no single method consistently performed best for all four biological systems.
The limitations of different methods allow scientists the chance for fundamental improvement.
"Deciphering gene networks is a very challenging problem because they are high dimensional and span multiple scales," Song said, "however, thinking to the availability of high-throughput biotechnologies probing into many facets of a cell, we are at an unprecedented time to tackle such problems with the help of powerful computers. These networks are expected to greatly enhance our understanding of molecular mechanisms in many biological systems, e.g., in metastatic cancer cells and advanced biofuel production."